Mining Heterogeneous Data with Cube Networks
Speaker
Carl Yang, University of Illinois, Urbana Champaign
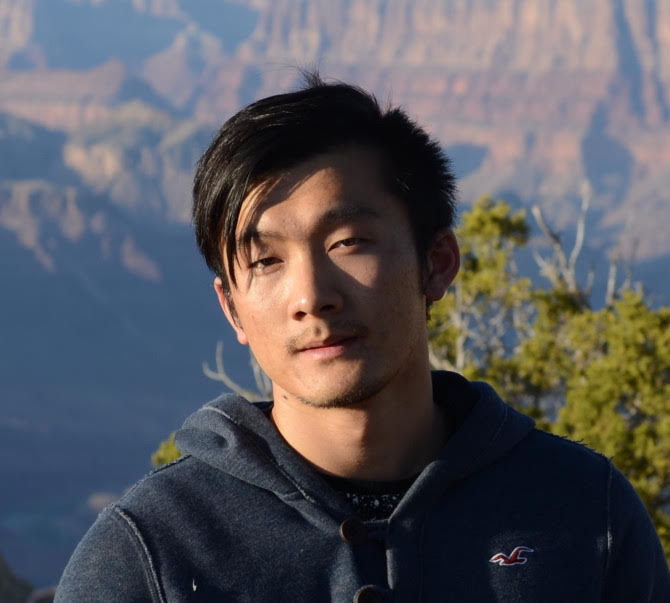
Time
2018-11-27 16:00:00 ~ 2018-11-27 17:30:00
Location
Room 3-517A, SEIEE Building, Shanghai Jiao Tong University
Host
Weinan Zhang, Assistant Professor, John Hopcroft Center for Computer Science
Abstract
Data are being generated everywhere in extraordinary speed and amount nowadays Due to advanced data warehousing techniques and the much effort put into knowledge acquisition, lots of data are stored with structures, ready to be modeled under the setting of heterogeneous networks However, data in the networks are still noisy and incomplete, and entities interact in complex ways Traditional network analyses are mostly focused on links and node attributes They are ineffective in leveraging complex features and inefficient in exploring various network structures, which results in a huge gap between structured data and real-world applications
In this talk, I will cover several pieces of my recent work, under the theme of mining heterogeneous data with cube networks Data cube is a conceptual structure from traditional data mining that organizes data according to their essential properties, while network provides a generic model for data interactions The co-modeling of real-world data by cube and network is thus a powerful and flexible framework towards various key problems in data processing, knowledge acquiring, as well as downstream applications In the first work, I will introduce recent results on combining data cube and reinforcement learning for efficient query-specific network construction It works as an example of the powerful cube network framework, and motivates our further research on this topic After that, I will introduce a few works on heterogeneous network modeling, where the data heterogeneity can be simply framed as particular dimensions in the cube network context A few works on cube networks with industrial data will also be briefly covered to demonstrate the utility of the framework towards real-world applications Finally, I will conclude with some future plans about learning with cube networks as well as ideas on general heterogeneous data mining through neural architectures
Bio
Carl Yang is a fifth-year Ph.D. student with Jiawei Han in Computer Science at University of Illinois, Urbana Champaign. He received his B.Eng. in Computer Science at Zhejiang University under Xiaofei He in 2014. In his research, he develops data-driven techniques and neural architectures for learning with complex heterogeneous network data. His interests span data mining, machine learning and statistics, with a focus on leveraging graph analysis and deep learning techniques, to a wide range of questions including information network construction, entity/relation profiling, pattern-based network embedding and etc. Carl’s research results have been published in top conferences like KDD, WWW, ICDE, ECML-PKDD, ICDM and ICML.