Multi-Agent Actor-Critic for Mixed Cooperative-Competitive Environments
Speaker
Dr. Yi Wu, University of California Berkeley
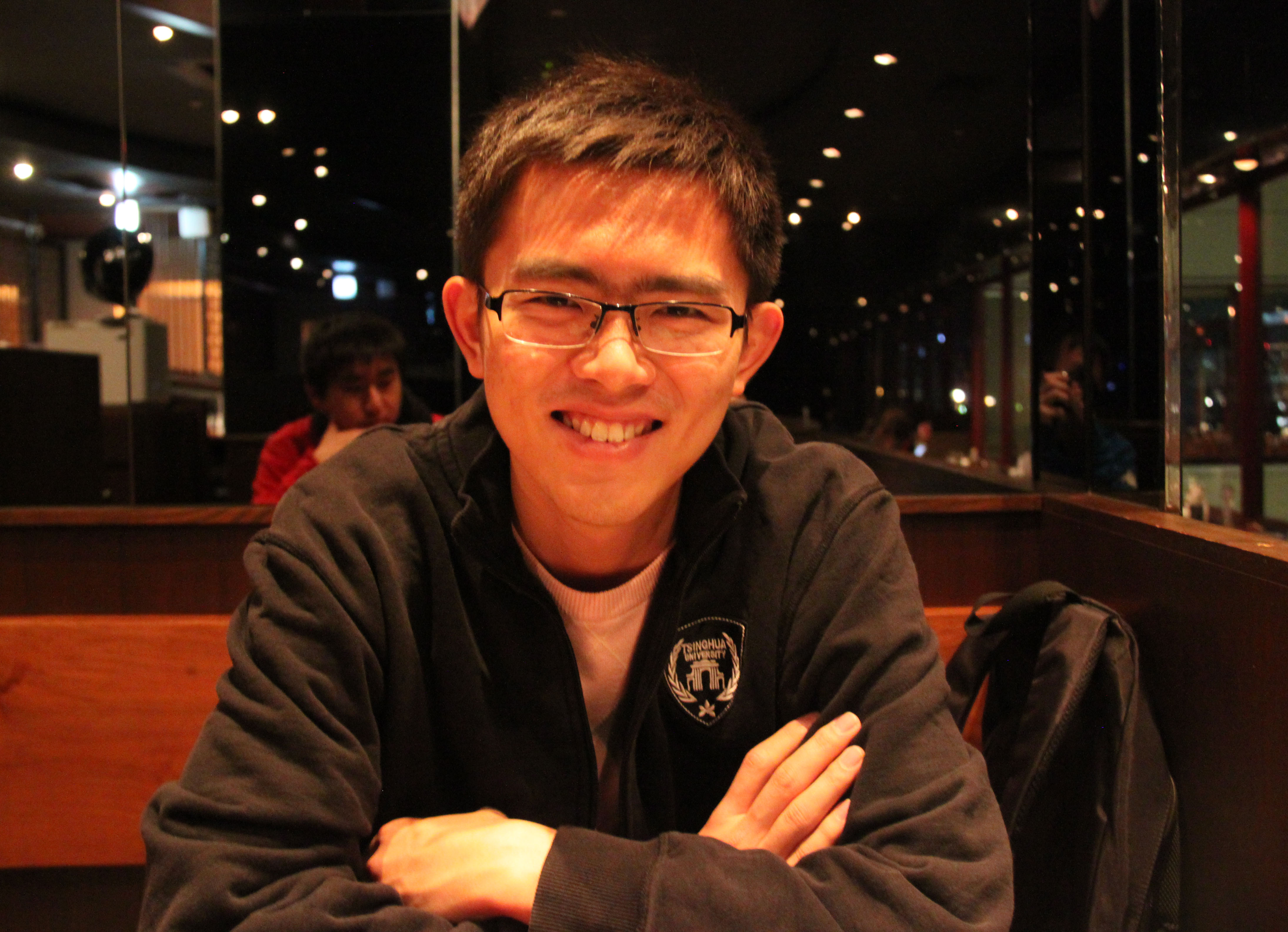
Time
2018-01-05 14:00:00 ~ 2018-01-05 15:30:00
Location
Room 208, Yifu Building
Host
Weinan Zhang
Abstract
We explore deep reinforcement learning methods for multi-agent domains We begin by analyzing the difficulty of traditional algorithms in the multi-agent case: Q-learning is challenged by an inherent non-stationarity of the environment, while policy gradient suffers from a variance that increases as the number of agents grows We then present an adaptation of actor-critic methods that considers action policies of other agents and is able to successfully learn policies that require complex multi-agent coordination Additionally, we introduce a training regimen utilizing an ensemble of policies for each agent that leads to more robust multi-agent policies We show the strength of our approach compared to existing methods in cooperative as well as competitive scenarios, where agent populations are able to discover various physical and informational coordination strategies
Bio
Yi Wu is now a 4th-year Computer Science Ph.D. at UC Berkeley advised by Prof. Stuart Russell. He received his B.E. from the special pilot class (Yao class) from Institute of Interdisciplinary Information Sciences, Tsinghua University. Yi's research focues on how to effectively incorporate human knowledge into AI models to produce both interpretable and generalizable solutions. He is now working on a variety of projects, including probabilistic programming, natural language processing and reinforcement learning. Besides research, Yi also won two medals in ACM/ICPC world finals and was a silver medalist in IOI.