Efficient Deep Imitation and Reinforcement Learning in Multi-Agent Environments
Speaker
Stephan Zheng, Caltech
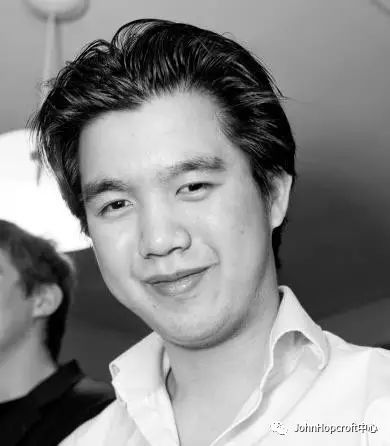
Time
2017-06-28 15:00:00 ~ 2017-06-28 16:00:00
Location
SEIEE-3-308
Host
Weinan Zhang
Abstract
Deep learning and reinforcement learning have been highly successful in training AI agents that perform low-level pattern recognition and short-term decision-making, such as image classification and playing the game of Go However, many practical problems, such as autonomous driving, intelligent logistics and robotics, require learning behavioral policies that are capable of high-level reasoning in complex environments
In this talk, I will present novel methods to learn policies for 2 challenges in this context: 1) reasoning over long timescales in spatiotemporal multi-agent games and 2) reasoning about cooperation in multi-agent coordination games In both settings, machine learning faces fundamental scalability and feasibility challenges To address these, I will present 2 novel deep imitation and reinforcement learning approaches
First, to learn long-term planning in multi-agent games, I will present a class of hierarchical deep learning models that operate on different timescales To illustrate their effectiveness, I will show that these models are able to learn to move like professional basketball players by imitation from human demonstrations
Second, I will demonstrate “MACE” (Multi-Agent Coordinated Exploration), a technique that improves the sample efficiency of reinforcement learning in games with many agents by jointly learning how to explore and coordinate
Finally, I will discuss ongoing research on improving long-term sequential prediction models via reinforcement learning and nonlinear Lyapunov control
Bio
Stephan Zheng (www.stephanzheng.com) is a Ph.D. candidate in the Machine Learning Group at Caltech, advised by Professor Yisong Yue. His main research focuses on developing new deep reinforcement learning methods for multi-agent environments. Previously, he has worked on deep imitation learning and robust deep learning. Stephan has published in leading machine learning and computer vision conferences, such as NIPS and CVPR. He was twice a research intern with Google Research and Google Brain. Before machine learning, he worked on theoretical high-energy physics and topological string theory. Stephan obtained his Master’s degrees in Mathematics and Theoretical Physics from the University of Cambridge and Utrecht University, and was a visiting student at Harvard University. He received the 2011 Lorentz prize in Theoretical Physics from the Royal Dutch Academy of Sciences and Arts, and twice the Dutch National Huygens Fellowship.