Scalable structure learning for spatiotemporal analysis
Speaker
Qi (Rose) Yu, University of Southern California
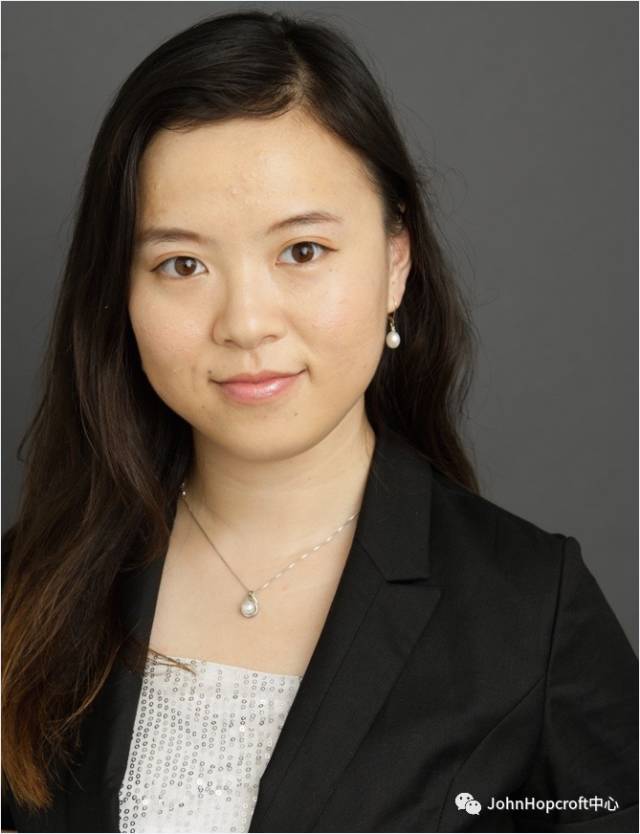
Time
2017-06-28 14:00:00 ~ 2017-06-28 15:00:00
Location
SEIEE-3-308
Host
Weinan Zhang
Abstract
Spatiotemporal data is ubiquitous in our daily life, including climate, transportation, and social media Today, data is being collected at an unprecedented scale As such, yesterday’s concepts and tools are insufficient to serve tomorrow’s data-driven decision makers Particularly, spatiotemporal data often demonstrates complex dependency structures and is of high dimensionality This requires new machine learning algorithms that can handle highly correlated samples, perform efficient dimension reduction, and generate structured predictions
In this talk, I will present a set of new machine learning tools for spatiotemporal data analysis, with an emphasis on structure learning I will discuss two frameworks
(1) Low-rank tensor learning Tensors provide natural representations for high-order correlations I will demonstrate scalable tensor methods for spatiotemporal analysis This includes a geometric rate and linear memory solver for low-rank tensor learning with theoretical guarantees
(2) Graph convolutional recurrent neural networks Many spatiotemporal problems are set in irregular geometry, such as non-grid graphs, which cannot be captured well by most existing deep learning methods I will present a novel deep architecture that can model irregular spatial temporal dependencies This model achieves significant improvements in traffic forecasting applications
The talk includes joint work with Yan Liu, Cyrus Shahabi, and Yaguang Li
Bio
Qi (Rose) Yu (www.roseyu.com) is a Ph.D. candidate, Annenberg fellow at the University of Southern California, focusing on machine learning and data analytics. She will join Caltech as a postdoc and then a tenure-track assistant professor in Northeastern University starting 2018 fall. Her research strives to develop machine learning methods to learn from large-scale spatiotemporal data, specifically in the domain of computational sustainability and social science. She has over a dozen publications in leading machine learning/data mining conferences (NIPS, ICML, AAAI, KDD), including best paper nominations. Previously, she was a visiting researcher in Stanford University. She has interned in various industrial research labs including IBM Watson, Yahoo, Intel Labs and Microsoft. As part of technology transfer, she co-founded a neighborhood discovery startup and led the team into the USC incubator program. She was nominated as one of the “2015 MIT Rising Stars in EECS”.