Learning Structured Representations for Natural Language
Speaker
Zhouhan Lin, the Mila lab of University of Montreal
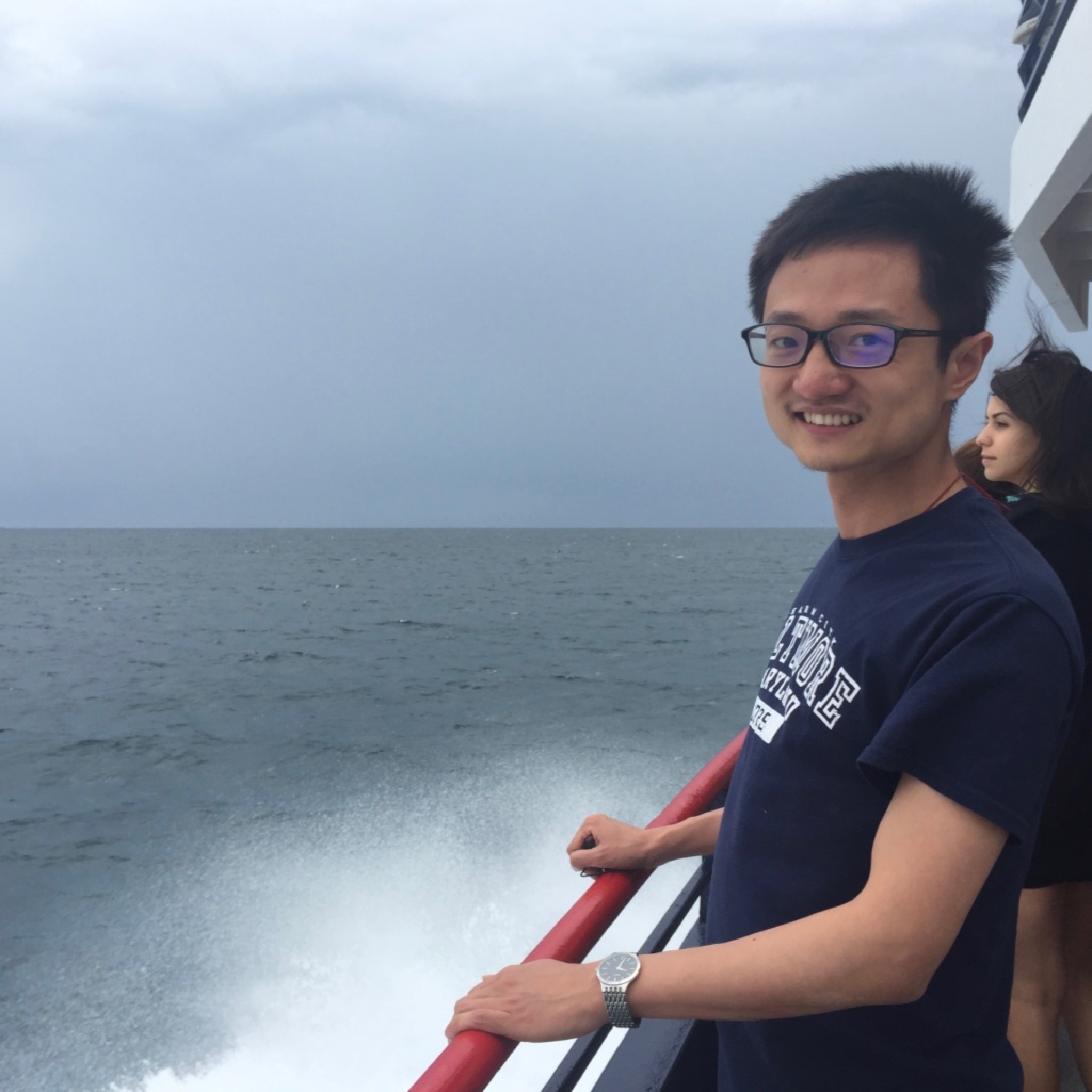
Time
2019-01-07 15:00:00 ~ 2019-01-07 16:30:00
Location
Room 3-412, SEIEE Building
Host
Jingwen Leng
Abstract
In this talk, we’ll cover several approaches in learning structured representations for natural language, which could benefit applications in various downstream tasks We’ll start from introducing a self-attentive sentence embedding, which aims at learning intra-sentence relation through attention mechanism and represent the semantics of a sentence in a matrix representation Then I’ll describe two models with the capability to learn richer structures in two different ways: a discretized way through reinforcement learning, and a softened way through backpropagation The discretized way is an adaptive neural network that reflects the language structure directly through the structure of the neural networks; while the softened way is a language model capable of learning implicit structures in the form of a binary tree Finally, I’ll show a sample application of the second application to a classical NLP task, which is syntactic parsing.
Bio
Zhouhan Lin is a Ph.D. student in his final year, under the supervision of Yoshua Bengio. He is from the Mila lab of University of Montreal, which is one of the three labs that initiates the resurgence of the neural network approach. Zhouhan’s current research is focused on the intersection of machine learning and natural language processing, including self-attention mechanisms, language models, parsers, QA systems, etc. Apart from NLP related topics, his research also covers binary and low precision neural networks, satellite image processing, etc.