Regularization Methods for Deep Learning
Speaker
Haizhao Yang, National University of Singapore
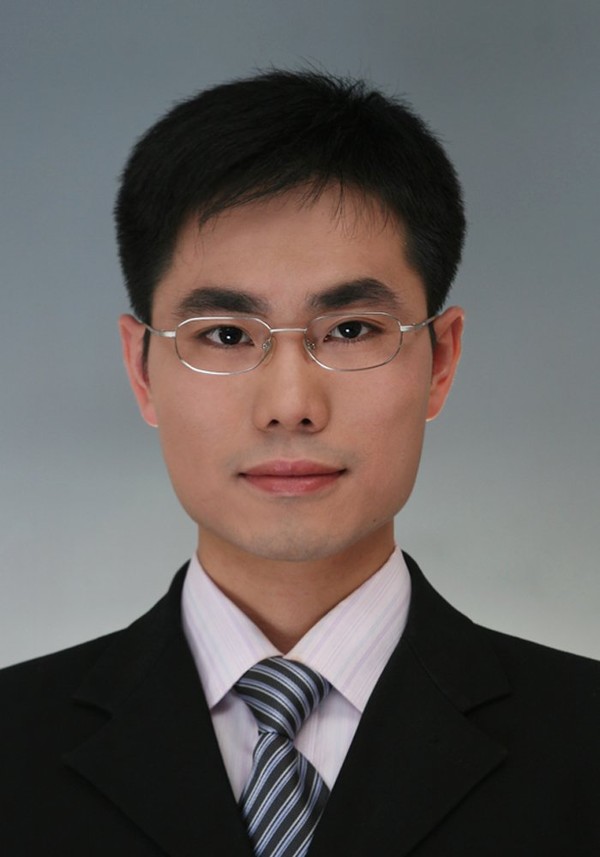
Time
2019-06-06 10:30:00 ~ 2019-06-06 11:30:00
Location
Room 3-412, SEIEE Building
Host
Jingwen Leng, Assistant Professor, John Hopcroft Center for Computer Science
Abstract
Overfitting frequently occurs in deep learning. In this talk, we introduce two novel regularization methods: Drop-Activation and DIA-Net, to reduce overfitting and improve generalization. The key idea of Drop-Activation is to weaken the nonlinear effect of ReLU activation functions by setting them to be identities randomly during training time; while the crucial idea of the DIA-Net is to enhance the communication of network attention via recurrent neural networks. Experimental results on CIFAR-10, CIFAR-100, SVHN, EMNIST, and ImageNet show that these regularization methods generally improve the performance of popular neural network architectures, and are harmonic with other regularization algorithms.
Bio
Dr. Haizhao Yang is currently an assistant professor in the Department of Mathematics and an affiliate of the Institute of Data Science at the National University of Singapore (NUS). Prior to joining NUS, Dr. Yang was a visiting assistant professor at Duke University from 2015 to 2017. Dr. Yang obtained his Ph.D. at Stanford University in 2015, M.S. at the University of Texas at Austin in 2012, and B.S. at Shanghai Jiao Tong University in 2010 all in applied mathematics.