Transparent Scaling of Deep Learning Systems through Dataflow Graph Analysis
Speaker
Jinyang Li, New York University
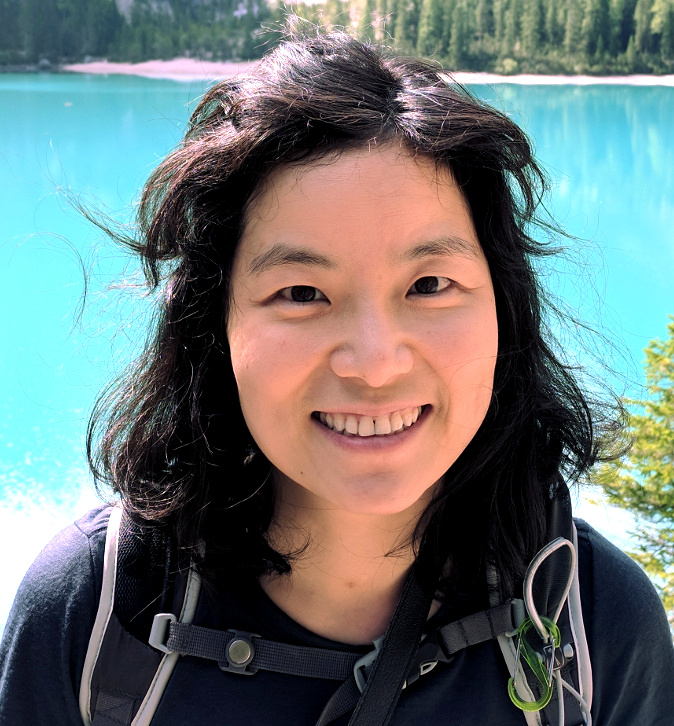
Time
2019-07-04 14:00:00 ~ 2019-07-04 15:30:00
Location
Room 3-412, SEIEE Building
Host
Weinan Zhang, Assistant Professor, John Hopcroft Center for Computer Science
Abstract
As deep learning research pushes towards using larger and more sophisticated models, system infrastructure must use many GPUs efficiently. Analyzing the dataflow graph that represents the DNN computation is a promising avenue for optimization. By specializing execution for a given dataflow graph, we can accelerate DNN computation in ways that are transparent to programmers. Inthis talk, I show the benefits of dataflow graph analysis by discussing two recent systems that we've built to support large model training and low-latency inference. To train very large DNN models, Tofu automatically re-writes a dataflow graph of tensor operators into an equivalent parallel graph in which each original operator can be executed in parallel across multiple GPUs. To achieve low-latency inference, Batchmaker discovers identical sub-graph computation among different requests to enable batched execution of requests arriving at different times.
Bio
Jinyang Li is a professor of computer science at New York University. Her research is focused on developing better system infrastructure to accelerate machine learning and web applications. Most recently, her group has released DGL, an open-source library for programming graph neural networks. Her honors include a NSF CAREER award, a Sloan Research Fellowship and multiple Google research awards. She received her B.S. from National University of Singapore and her Ph.D. from MIT, both in Computer Science.