Smooth Contextual Bandits: Bridging the Parametric and Non-differentiable Regret Regimes
Speaker
Xiaojie Mao,Cornell University
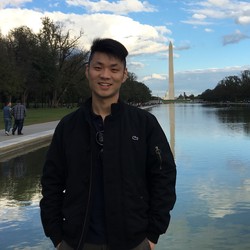
Time
2020-01-02 10:30:00 ~ 2020-01-02 12:30:00
Location
Room 1319, Software Expert Building
Host
Shuai Li, Assistant Professor, John Hopcroft Center for Computer Science
Abstract
We study a nonparametric contextual bandit problem where the expected reward functions belong to a Hölder class. We show how this interpolates between two extremes that were previously studied in isolation: non-differentiable bandits, where rate-optimal regret is achieved by running separate non-contextual bandits in different context regions, and parametric-response bandits, where rate-optimal regret can be achieved with minimal or no exploration due to infinite extrapolatability. We develop a novel algorithm that carefully adjusts to all smoothness settings and we prove its regret is rate-optimal by establishing matching upper and lower bounds, recovering the existing results at the two extremes. In this sense, our work bridges the gap between the existing literature on parametric and non-differentiable contextual bandit problems and between bandit algorithms that exclusively use global or local information, shedding light on the crucial interplay of complexity and regret in contextual bandits.
Bio
Xiaojie Mao is a PhD candidate in Department of Statistics and Data Science at Cornell University, and is currently based in Cornell Tech campus in New York City. He researches at the intersection of causal inference and statistical machine learning. He is particularly interested in developing flexible and robust statistical methods for data-driven decision-making that involves causal reasoning. See https://xiaojiemao.github.io/ for more information.