Unlocking Text Generation
Speaker
Junxian He, Carnegie Mellon University
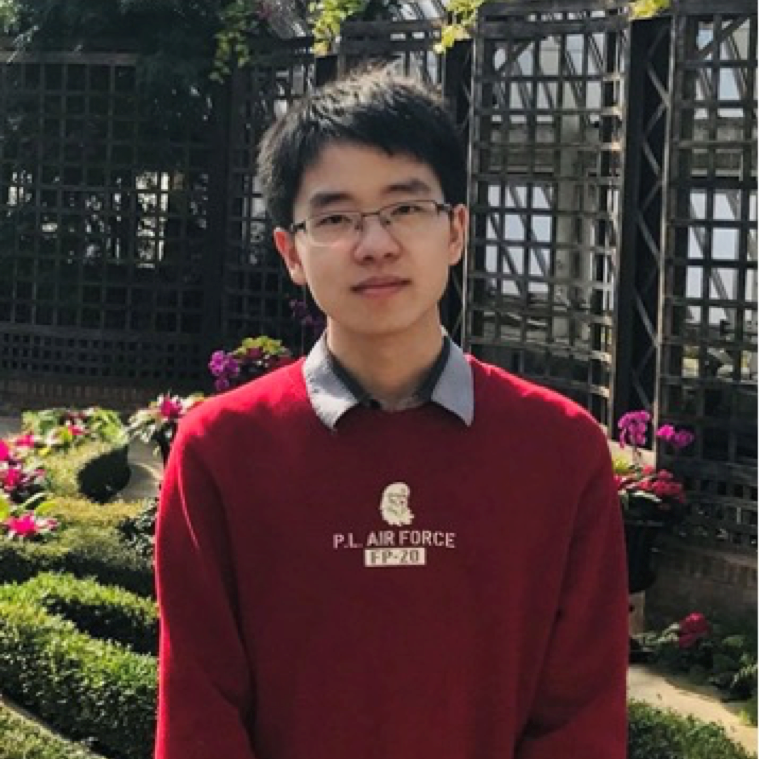
Time
2021-05-01 10:00:00 ~ 2021-05-01 11:30:00
Location
电信群楼1-418A会议室;ZOOM视频会议(会议号:678 378 53819 ,会议密码:832118)
Host
金海明
Abstract
Humans generate text in a way that involves latent structures and hidden factors, such as syntax, topicality, sentiment, prototype, etc. However, most machine learning systems deal with these hidden factors indirectly in an uninterpretable manner — deep neural networks often function like a blackbox. Thus it has been difficult to automatically analyze language, understand how the text is generated, and further control its generation. In this talk, I will cover my research efforts towards addressing these challenges as: (1) latent-variable models on deciphering the hidden factors of text; (2) controllable text generation including text style transfer, prototype-based text generation, and controllable text summarization; and (3) efficient non-parametric language models that generate text with an interpretable datastore. At the end of this talk I will briefly describe my visions on future work.
Bio
Junxian He is a fourth-year Ph.D. student in the school of computer science at Carnegie Mellon University, co-advised by Prof. Graham Neubig and Prof. Taylor Berg-Kirkpatrick. His research focuses on generative modeling of natural language, specifically on controllable text generation, latent-variable text modeling, and non-parametric text models. Before joining CMU, he obtained the bachelor degree from Shanghai Jiao Tong University. He is one of the ten recipients of 2020 Baidu PhD fellowship.