Online Policy Optimization for Robust MDP
Speaker
Baoxiang Wang, The Chinese University of Hong Kong(Shenzhen)
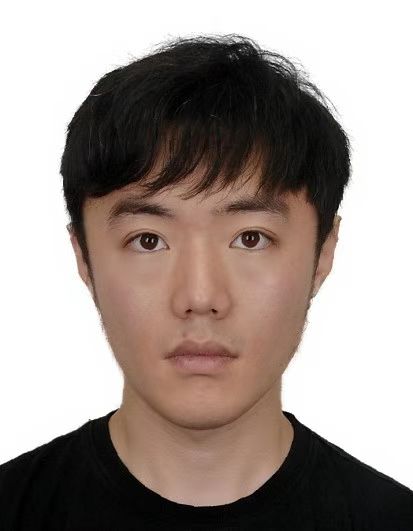
Time
2023-04-13 11:00:00 ~ 2023-04-13 12:00:00
Location
电信群楼3-414会议室
Host
李帅
Abstract
Reinforcement learning (RL) has exceeded human performance in many synthetic settings such as video games and Go. However, real-world deployment of end-to-end RL models is less common, as RL models can be very sensitive to slight perturbation of the environment. The robust Markov decision process (MDP) framework -- in which the transition probabilities belong to an uncertainty set around a nominal model -- provides one way to develop robust models. While previous analysis shows RL algorithms are effective assuming access to a generative model, it remains unclear whether RL can be efficient under a more realistic online setting, which requires a careful balance between exploration and exploitation. In this work, we consider online robust MDP by interacting with an unknown nominal system. We propose a robust optimistic policy optimization algorithm that is provably efficient. To address the additional uncertainty caused by an adversarial environment, our model features a new optimistic update rule derived via Fenchel conjugates. Our analysis establishes the first regret bound for online robust MDPs.
Bio
Baoxiang Wang is an assistant professor in School of Data Science, The Chinese University of Hong Kong, Shenzhen. Baoxiang works on the broad area of reinforcement learning and reinforcement learning theory. He obtained his Ph.D. in Computer Science and Engineering from The Chinese University of Hong Kong in 2020 under Siu On Chan and Andrej Bogdanov. Before that, he obtained his B.E. in Information Security from Shanghai Jiao Tong University in 2014. He publishes works in machine learning conferences like ICML, NeurIPS, ICLR, and theory venues like ITCS.