A Definition of Non-Stationary Bandits
Speaker
Yueyang Liu, Stanford University
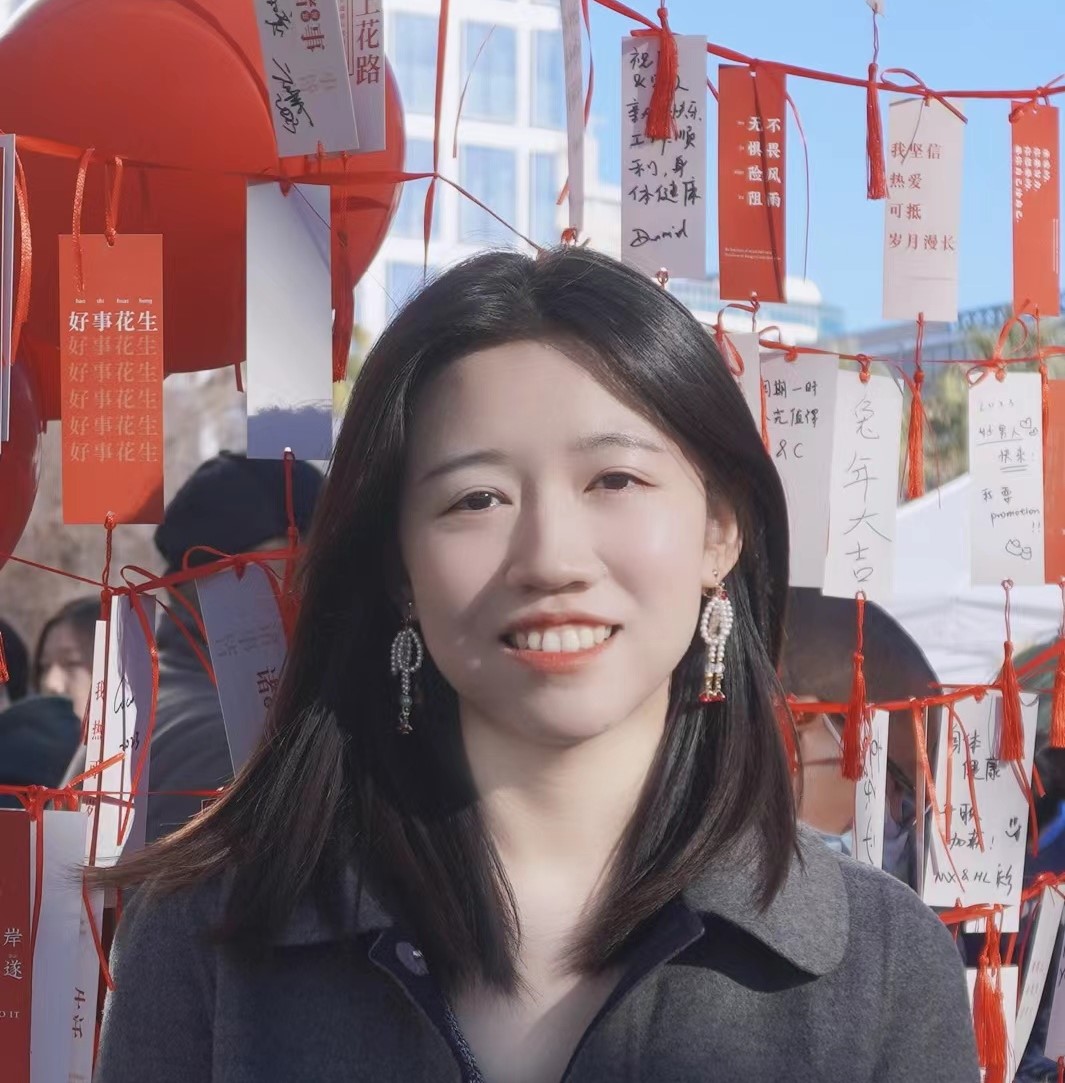
Time
2023-07-03 15:00:00 ~ 2023-07-03 16:30:00
Location
电信群楼3-404会议室
Host
李帅
Abstract
Despite the subject of non-stationary bandit learning having attracted much recent attention, we have yet to identify a formal definition of non-stationarity that can consistently distinguish non-stationary bandits from stationary ones. Prior work has characterized non-stationary bandits as bandits for which the reward distribution changes over time. We demonstrate that this definition can ambiguously classify the same bandit as both stationary and non-stationary; this ambiguity arises in the existing definition's dependence on the latent sequence of reward distributions. In addition, the definition has given rise to two widely used notions of regret: the dynamic regret and the weak regret. These notions are not indicative of qualitative agent performance in some bandits that are intuitively nearly-stationary, i.e., non-stationary bandits that closely resemble stationary ones. In addition, this definition of non-stationary bandits has led to the design of agents that explore excessively. We introduce a formal definition of non-stationary bandits that resolves these issues. In addition, for two bandits that provide agents with indistinguishable experiences, our definition classifies them as both stationary or both non-stationary. Our definition also applies seamlessly to both the Bayesian and the frequentist formulations of bandits, providing a unified approach.
Bio
Yueyang Liu is a fifth-year PhD candidate at Stanford University, where she is part of the Operations Research group in the Department of Management Science and Engineering. Her interest lies in continual learning. She is advised by Benjamin Van Roy and Kuang Xu.