The Smoothed and Semi-Random Possibilities of Social Choice
Speaker
Lirong Xia, Rensselaer Polytechnic Institute (RPI)
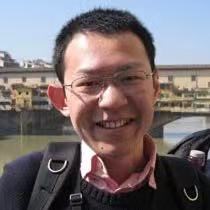
Time
2023-08-22 10:45:00 ~ 2023-08-22 11:45:00
Location
软件大楼专家楼1319会议室
Host
陶表帅
Abstract
Social choice studies how to aggregate agents' preferences to make a collective decision. A major obstacle in designing desirable social choice mechanisms is the wide presence of worst-case paradoxes and impossibility theorems. While there is a large body of literature on using average-case analysis to circumvent the impossibilities, the models in previous work were criticized for being unrealistic, and technical tools for going beyond a few voting rules and a few distributions are lacking.
We take a worst average-case approach to propose a natural, general, and more realistic semi-random model that resembles the celebrated smoothed analysis. We characterize the conditions and rates for the semi-random likelihood of Condorcet's paradox, the ANR impossibility theorem, and the Gibbard-Satterthwaite theorem to vanish by developing a "polyhedral approach" and thus addressing several long-standing open questions under more general models. Our model and results illustrate the smoothed and semi-random possibilities of social choice, which help establish a more realistic foundation of social choice beyond the worst cases.
The talk is based on the following papers:
https://arxiv.org/abs/2006.06875
https://arxiv.org/abs/2011.03791
https://arxiv.org/abs/2202.06411
Bio
Lirong Xia is an associate professor in the Department of Computer Science at Rensselaer Polytechnic Institute (RPI). He was an NSF CI Fellow at the Center for Research on Computation and Society at Harvard University. He received his Ph.D. in Computer Science and M.A. in Economics from Duke University. His research focuses on the intersection of computer science and microeconomics. He is the recipient of an NSF CAREER award, a Rensselaer James M. Tien'66 Early Career Award, and was named as one of "AI's 10 to watch" by IEEE Intelligent Systems.