Strong Spatial Mixing for Colorings on Trees and its Algorithmic Applications
Speaker
Zongchen Chen, University at Buffalo
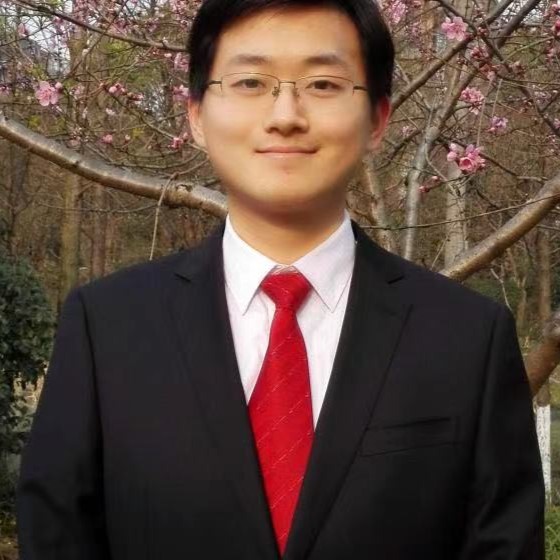
Time
2023-12-06 15:01:00 ~ 2023-12-06 15:01:00
Location
电信群楼3号楼326A
Host
杨宽
Abstract
Strong spatial mixing (SSM) is an important quantitative notion of correlation decay for Gibbs distributions arising in statistical physics, probability theory, and theoretical computer science. A longstanding conjecture is that the uniform distribution on proper q-colorings on a D-regular tree exhibits SSM whenever q >= D+1. Moreover, it is widely believed that as long as SSM holds on bounded-degree trees with q colors, one would obtain an efficient sampler for q-colorings on all bounded-degree graphs via simple Markov chain algorithms. We show SSM for q-colorings on all trees of maximum degree D whenever q >= D+3, almost fully resolving the first conjecture. Towards sampling, we establish optimal mixing of the Glauber dynamics for q-colorings on all graphs of maximum degree D and sufficiently large girth whenever q >= D+3. Joint work with Kuikui Liu, Nitya Mani, and Ankur Moitra.
Bio
Zongchen Chen is an assistant professor in the Department of Computer Science & Engineering at University at Buffalo. Previously, he spent two years as an instructor (postdoc) in the Department of Mathematics at MIT. He received the PhD degree in Algorithms, Combinatorics and Optimization (ACO) from the School of Computer Science at Georgia Tech in 2021. He has broad interests in randomized algorithms, discrete probability, and machine learning. Currently, his research focuses on Markov chain Monte Carlo (MCMC) methods, approximate counting and sampling, and learning and testing of high-dimensional distributions.