Machine Learning in Finance: Uncertainty Quantification, Generative Learning, Model Stability, and Their Applications
Speaker
Xing YAN,Renmin University of China
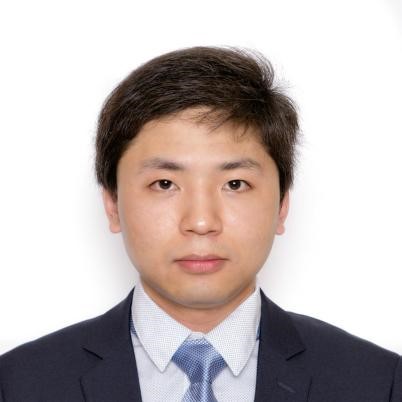
Time
2024-05-31 16:00:00 ~ 2024-05-31 17:30:00
Location
上海交通大学电信群楼3-404会议室
Host
李帅
Abstract
AI in Finance is a fascinating cross-disciplinary area. However, a significant gap exists between these two domains, characterized by distinct cultures and objectives. In this presentation, our goal is to bridge this gap by highlighting the unique aspects of machine learning in finance and introducing novel methodologies with successful applications. We will cover three key topics: uncertainty quantification, generative learning, and model stability in finance. We contend that end-to-end black-box models are generally ineffective in this context. Instead, models elaborately designed to capture the natures of financial data, such as uncertainty, dependency structures, tail properties, and temporal variation, are more likely to succeed. The methodologies we have proposed achieve state-of-the-art performance in problems such as risk management, portfolio construction, and derivatives pricing.
Bio
Dr. Yan obtained his Ph.D. degree from Department of SEEM, CUHK in 2019. He also received a master's degree in computer science from Institute of Computing Technology, Chinese Academy of Sciences, and a bachelor's degree in pure mathematics from Nankai University. Dr. Yan works at the intersection of AI and finance/business. He is interested in topics such as tail risk management, empirical asset pricing, portfolios, derivatives, consumer credit, etc. Recently, he is also interested in OOD generalization, uncertainty quantification, etc. in machine learning. His research works have been published in both machine learning journals/conferences and finance journals, such as IEEE TPAMI, IEEE TNNLS, European Journal of Operational Research, Journal of Economic Dynamics and Control, NeurIPS, AAAI, ECCV, ICAIF, etc.