Differential Privacy for Modern Deep Learning
Speaker
王帝,沙特阿拉伯阿卜杜拉国王科技大学
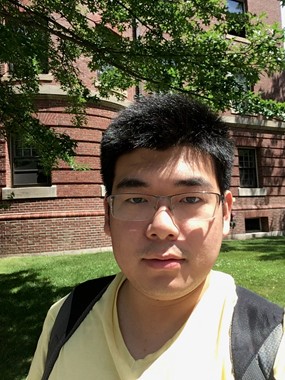
Time
2024-06-11 13:30:00 ~ 2024-06-11 15:30:00
Location
上海交通大学软件大楼专家楼1319会议室
Host
向立瑶
Abstract
Differential Privacy (DP) is rapidly becoming the gold standard for private data analysis. In recent years, a substantial body of research has emerged, proposing a diverse array of differentially private training algorithms tailored to various deep learning models. In this talk, I will present our recent work on DP for modern deep learning architectures. First, I will focus on private and Byzantine-resilient Federated Learning algorithms. Second, I will introduce a series of DP training algorithms designed for Graph Neural Networks (GNNs), which can preserve node-level privacy. Finally, I will showcase our latest work on privacy auditing for private hyper-parameter selection in DP Stochastic Gradient Descent.
Bio
王帝,博士生导师,目前是沙特阿拉伯阿卜杜拉国王科技大学计算机系助理教授和统计系兼职教授。他同时担任可信智能与数据分析实验室(Provable Responsible AI and Data Analytics (PRADA) Lab)主任,KAUST计算生物研究中心成员,和SDAIA-KAUST数据科学与人工智能中心兼职教授。他曾访问波士顿大学,哈佛大学,西蒙斯理论计算研究院和加州大学伯克利分校,并且是芬兰赫尔辛基大学和芬兰人工智能中心客座教授。王帝教授于2020年在美国纽约州立大学布法罗分校取得计算机与工程博士学位。在此之前,他于2015年在加拿大西安大略大学取得数学硕士学位,2014年在山东大学取得数学与应用数学学士学位。王帝教授目前的研究领域包括可信机器学习,大模型,科学智能等。他已经以第一作者和通讯作者在机器学习,网络安全,数据库和理论计算机顶级期刊和会议例如ICML,NeurIPS,ICLR, JMLR,S&P,USENIX,IEEE Transactions on Information Theory,PODS,SIGMOD, VLDB等发表超过50篇论文,并且曾获得多个会议最佳论文奖项。他多次担任国际顶级学术会议和期刊的领域主席和审稿人,并且多次受邀于国际顶级学术会议和学术机构做报告。