Unsupervised Adaptation to Online Label Shift
Speaker
Ruihan Wu, University of California, San Diego
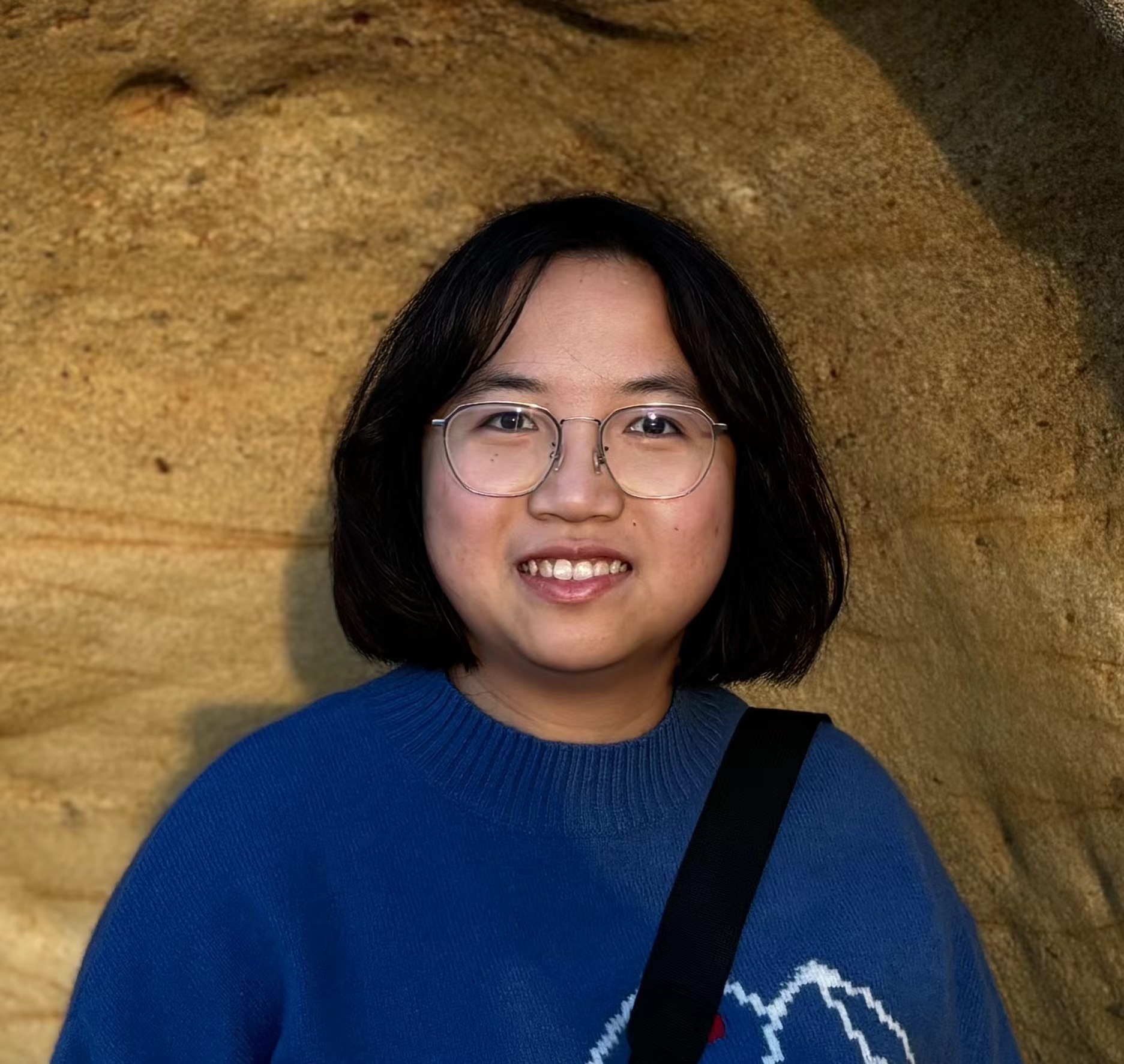
Time
2024-07-12 14:00:00 ~ 2024-07-12 15:00:00
Location
上海交通大学电信群楼3-412会议室
Host
梁诗宇
Abstract
Machine learning models often encounter distribution shifts when deployed in the real world. In this talk, I will focus on adaptation to label distribution shift in the online setting, where the test-time label distribution is continually changing and the model must dynamically adapt to it without observing the true label. Leveraging a novel analysis, I will show that the lack of true label does not hinder estimation of the expected test loss, which enables the reduction of online label shift adaptation to conventional online learning. The algorithm has the theoretical guarantee and is empirically effective as evaluated. I will further introduce how utilize the self-supervised learning to refine the feature extraction process. The algorithm with online feature updates further improves the performance while still holding the theoretical guarantee.
Bio
Ruihan Wu is a postdoctoral researcher at the University of California, San Diego. Her research interests mainly lie in machine learning, with a recent focus on privacy-preserving machine learning, machine learning safety, and practical applications of online and bandit algorithms. She received her Ph.D. from Cornell University in 2023 and her B.E. from Tsinghua University in 2018. She received the LinkedIn PhD Fellowship in 2022.